Our paper entitled “CTS: Concurrent Teacher-Student Reinforcement Learning for Legged Locomotion” is accepted by RA-L 2024.
In this paper, we propose CTS, a novel Concurrent Teacher-Student reinforcement learning architecture for legged locomotion over uneven terrains. Different from conventional teacher-student architecture that trains the teacher policy via RL first and then transfers the knowledge to the student policy through supervised learning, our proposed architecture trains teacher and student policy networks concurrently under the reinforcement learning paradigm. To this end, we develop a new training scheme based on a modified proximal policy gradient (PPO) method that exploits data samples collected from the interactions between both the teacher and the student policies with the environment. The effectiveness of the proposed architecture and the new training scheme is demonstrated through substantial quantitative simulation comparisons with the state-of-the-art approaches and extensive indoor and outdoor experiments with quadrupedal and point-foot bipedal robot platforms, showcasing robust and agile locomotion capability. Quantitative simulation comparisons show that our approach reduces the average velocity tracking error by up to 20% compared to the two-stage teacher-student, demonstrating significant superiority in addressing blind locomotion tasks.
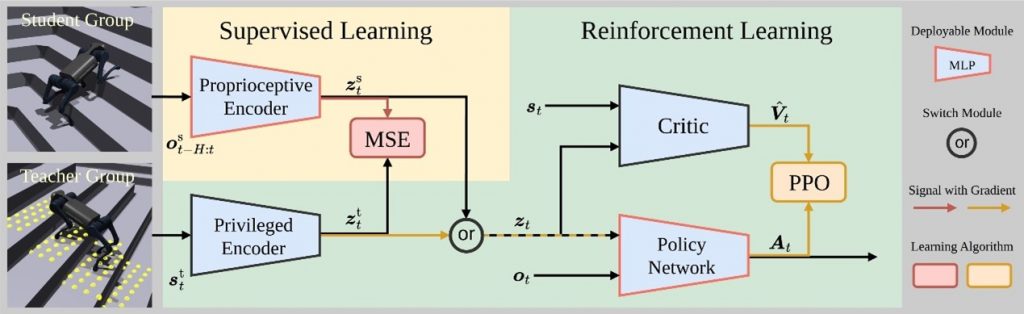